Release date: March 16, 2022
Catalogue number: 45-20-0003
ISSN: 2816-2250
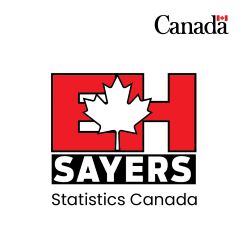
Canada’s diversity and rich cultural heritage have been shaped by the people who have come from all over the world to call it home. But even in our multicultural society, eliminating all forms of discrimination remains a challenge. In this episode, we turn a critical eye to the ways that cognitive bias risks perpetuating systemic racism. Statistics are supposed to accurately reflect the world around us, but are all data created equal? Join us as we explore the role data can play to make Canada a more equal society for all.
Host
Tegan Bridge
Guests
- Sarah Messou-Ghelazzi, Communications Officer, Statistics Canada
- Filsan Hujaleh, Analyst, Centre for Social Data Insights and Innovation, Statistics Canada
- Jeff Latimer, Director General, accountable for Health, Justice, Diversity and Populations, Statistics Canada
Listen to audio
Eh Sayers Episode 6 - Created Equal - Transcript
Sarah: The initial idea for the podcast on systemic racism came to me after observing everything that has, that happened at the beginning of the pandemic in 2020. The march in Ottawa, where the Prime Minister took a knee, everything that happened around the world with George Floyd. I think it removed a lot of the blinders that I personally had as a person of color. I thought it would be a nice way to say, “this actually exists”, and it's nice that we should be aware of it and we should as a group and as individuals, make a decision to make things better.
(Theme)
Tegan: Welcome to Eh Sayers, a podcast from Statistics Canada, where we meet the people behind the data and explore the stories behind the numbers. I’m your host, Tegan. Today's topic was suggested by my colleague, Sarah.
Sarah: So, my name is Sarah Messou-Ghelazzi and I'm a Junior communications officer for STATCAN.
Tegan: Do you notice any difference now a year and a half or so past summer of 2020?
Sarah: So, past summer 2020, I do see that there is a difference, at least in the way we talk about racism in society. I think it's no longer a taboo where people would get offended if we talk about racism. I think a lot more people are willing to be allies. They are wanting to join the conversation. They're willing to do their best to make changes at a personal level, I think.
Tegan: What do you hope listeners would get out of or learn from an episode about systemic racism and data?
Sarah: Data do tell a story. And with data we can do a lot. My takeaway is that we, as the people, take this data and try to change our approach and try to change our views of people of color, at least visible minorities. Because it is great to have all this data and it is great to have awareness. Let's take a step further. Let's see how this impacts communities, which means millions of people at the end of the day and how we can do better, how we can be more inclusive. I think the past 18 months, almost two years we've had awareness, we have had information and we will continue to have information. Let's take this information and turn it into action.
Tegan: Thank you, Sarah, for the great suggestion. Systemic racism, let’s talk about it.
Tegan: Could you please introduce yourself?
Filsan: My name is Filsan Hujaleh and I'm an analyst with the Centre for Social Data Insights and Innovation at Statistics Canada.
Tegan: And what do you do at StatCan?
Filsan: I do research, you know that's related to racialized groups. More specifically, more like ethnocultural immigration type of research. So it's different population groups, but more or less looking at issues that have to do with racialization inclusion and exclusion, and the different experiences that diverse groups that have, specially those with intersecting identities.
Tegan: Could you help us understand what systemic racism is?
Filsan: OK, so the Ontario Human Rights Commission defines find systemic discrimination as patterns of behaviour, policies or practices that are part of the structures of an organization and which create or perpetuate disadvantage for racialized persons.
Systemic racism, institutional racism, or I think, are, like you know behaviors or attitudes that are embedded within institutions set or a barrier to different racialized groups, right? So it can manifest itself in different ways, and I think what it boils down to is, there is a system in place, and it was designed for a particular group of people. We can take examples, for example if you look at a refugee to Canadian society whose from a non-European country, right? So when a person comes to Canadian society they kind of have to learn the institutions and how to navigate them and there could be barriers such as, you know, language barriers and not knowing the culture of a particular place or institution. Simple things like not knowing how to fill out a form. Also, the system not serving that person needs. So not being able to access particular services because of a barrier, it can be an environmental barrier. It can be, you know, the physical, actual access to a place. So it can be so many different things but always thinking about it of like you know in terms of needs, not being met and those barriers are, I think some ways to kind of understand systemic exclusions.
Tegan: Do you have a specific example that you’d like to talk about?
Filsan: So if we use the example of, you know Black youth and navigating different systems that are interconnected, right? So if you look at the education system, do they have the support outside and can this system support them? Or like do they see themselves in the curriculum? You know, are they more surveilled? You know, in schools? Are there behaviors, like are they expected to act and dress a certain way that this norm, and that they don't fit this norm, right? Are they streamed, are there exclusionary practices that happen in the school? That's one way, right to look at it, and then if you look outside of that, if they walk down the street are black youth more likely to again be surveilled and to be checked? And they're not allowed to kind of move freely in society, that they have a police officer coming up to them to ask any questions or carding them, for instance. Are they more likely to be stopped while driving a car? So that's another way of, you know, being not being able to kind of move freely in public spaces. And that also contributes to them, you know, having more contact with the justice system if they're over policed, for instance. If you go to hospitals and stuff, are their other concerns can be taken seriously? Yeah, they're going to be provided the care that they need to a standard that is acceptable. So as you can see, like in every domain, in social life. Whether that's, you know, the law or health or the schooling education system, it's these behaviors that you will see that are exclusionary towards different population groups, which are barriers and which make it very difficult for people to move freely. And to, you know, reach their full potential even.
If you'd like to learn more about this and the complexities around collecting data on racialized groups and their experiences, Jeff Latimer would be a great person to speak to.
Jeff: I'm Jeff Latimer, Director General, accountable for Health, Justice, Diversity and Populations at Statistics Canada.
Tegan: There's this idea that numbers don't lie. Why do people believe that all data are neutral and objective? And what are some of the problems that come with this belief?
Jeff: Yeah, I'm not convinced that all data are equal, so let me start by saying that's, in my opinion, a myth. I'll try and explain it in a relatively simple way. At Statistics Canada, let's just start with our data and I think that's a great way to start. So, we collect two different types of data. Basically we collect data from administrative systems, like a hospital admissions or police reported crime. Those data are great. They're all there, that’s 100% of every single crime that's reported to police is there, but it's not a measure of all crime in Canada. It's a measure of police reported crime because not all crime is reported to police. That's an example of how there's bias that you tried to use our police reported crime as a measure of all crime you would be mistaken.
In surveys, which is the other way we collect information. We talk to Canadians. We ask them questions. We need to make sure that the group of Canadians we are asking is generally representative of the population as a whole, and it's quite difficult to ensure your surveys are representative of the entire population. And so data that doesn't necessarily ensure you have the entire database in mind or the entire universe or that doesn't necessarily ensure you have a representative sample of the population will not necessarily be as accurate as if you do. It doesn't mean they are not accurate, but the likelihood of them being biased or inaccurate is quite high, so there's many ways of collecting information you can start with asking a group of let's just say on the street. You can walk down the street and ask a group of Canadians. What do you think about XY or Z? You may have a representative sample, but highly unlikely, particularly if you're standing outside of, I don't know, the Green Party convention and you're asking about environment. Now, the people that are walking by you are going to be much more likely to give you an answer in relation to the Green Party's positions on something.
So, bias is so critical and not all data is equal. The best data is those that are collected with the with all the protections in place to reduce the bias as much as possible, so that's very different than data collected without, with bias.
Tegan: What steps does Statistics Canada take to avoid bias?
Jeff: I'll only talk about our surveys. I think that's really the most important aspect. We collect information from Canadians in all areas of Statistics Canada, from labour to health, justice, etc. And the first thing we do is we ensure that the sampling, so in other words, how we pick the people to respond to us is as close as possible to the population that it represents those that we want to speak to. But at the same time. If not everybody will respond back to us, and so there's going to be still some potential bias, even though we've selected the right people, not all of them will respond, so we have to adjust our responses to ensure that the people we've spoken to generally speak on behalf of all Canadians. And so we have, you know, we have statistical methods of what we call weighting the data, so some responses will be given more value than other responses, so that when we calculate something. It's generally as accurate as possible, so those are two ways that we try and avoid bias.
Tegan: So what information does StatCan gather on race and ethnicity on the census?
Jeff: Yeah, so the first thing to clarify is that the census goes out to every household in Canada, but we only ask race and ethnicity questions one out of every four households. We do that though in order to be able to provide accurate estimates of the diversity of the population.
So for those households where we do ask race and ethnicity, we asked them to self-identify what group they believe they were, that they belong to and we offer them a series of 10 different choices. If we don't necessarily use the term race because for a lot of people race is a social construct and so we ask individuals in this country to self-identify the term that best describes them. Well, we also have subsequent questions beyond the simple population groups to look at ethnicity and where they're born, and immigration status and a number of other variables that we can combine to get a better picture of the country as a whole. And you know, having these race and ethnicity data on the census is so critical if you think about, you know, we want our institutions we want to, we want the policies and programs to reflect or mirror the population. And without. Understanding you know the composition or diversity of the population, we really would struggle with looking at those issues and so even though it's only on one out of every four.
Tegan: That is, one of every four households.
We’re able to calculate the population we are able to estimate the population of individuals, say black Canadians or South Asian Canadians by using the census data.
Tegan: Why is it so important to have high quality data in the context of systemic racism?
Jeff: Oh that's great question. So yeah, it really is because I think there's lots of data out there. As you know their data and not all data is created equal and I think it's critical that we understand, you know, we understand the different experiences and the different outcomes of all Canadians and all individuals in Canada. And so, if you think about it, racism as a construct is very hard to measure. But we can measure experiences and outcomes. So let me give you an example. Just I'll pick something quite simple. If we wanted to look at individuals who are overqualified in Canada, it's a very simple idea. Generally, we'll say if an individual is has a university degree but is in a job that only requires high school, we would consider them to be over qualified. When we look at that variable, we see about 15-14% of Canadians would be considered over qualified. But when we delve deeper and we start to disaggregate that statistic and we look at let's say immigrants in Canada, that number goes from 14 all the way to 22%. And if we look within the immigrant category and look at racialized immigrants, that jumps up to 26% and you can start to see. OK, maybe there's I'm not suggesting there are, but maybe there's some systemic racist issues and you have to be your data has to be really critical for you to be able to answer that question.
We could even go further with the racialized immigrants and just look at one of those ten categories of populations and we'll see 69% of Filipino immigrants in Canada are over qualified. Now, if that data is not reliable and it is not constructed very well without bias, then those numbers will not be meaningful, and so it's really critical that the data we collect can reflect the outcomes and experiences of all Canadians.
Tegan: How can data be misused perpetuate harmful stereotypes?
Jeff: This this is such an important topic for me. Uh, I'm responsible for the disaggregated data action plan at Statistics Canada with my colleague Tom Dufour. Everyone asked, what's the biggest risk and disaggregating data, and I would argue one of the biggest risks is further stigmatizing individuals when the data is really there to identify inequalities and inequities in society. So I'll give you a really good example; right now we're considering with the chiefs of police across the entire country to add to our, our police reported crime statistics, indigenous identity and racialized identity. Right now in Canada we cannot tell you the proportion of indigenous or racialized groups that come in contact with the police.
Now these data, once we're successful in collecting these data, we're going to for the first time, be able to talk about X proportion of black Canadians or South Asian Canadians have come in contact with the police, and that's not necessarily suggesting that they're more likely to commit criminal behaviour, or that they're more criminogenic. It just means that they have come into contact with the police, and there's very many sociological, you know, explanations for why that may or may not be true, including unemployment rates in education and over policing and communities and income and all sorts of reasons.
So, those data, while they could be misused, they theoretically should be used to identify some systemic issues that may be occurring in society. So, I think it's like I would like to call it a double edged sword. I do believe that it is worth the risk to count, to collect and to analyze these data so we can identify inequities and we need to do it though in a very careful and well thought out manner.
Tegan: You said disaggregated data. What is disaggregated data?
Jeff: So disaggregated data is simply a way of analyzing the data that goes deeper. So normally, for example, we could give you, for example, I could tell you the unemployment rate of Canadians and then I could tell you the unemployment rate of men and women. I've started to disaggregate. Break it apart into two categories, but that's generally what we call a binary disaggregation. It's not that helpful. It's helpful to understand male and females. But if I were to give you non visible minority and visible minorities, that doesn't really tell you very much because visible minorities are not a homogeneous group. They are very they’re varied and so to disaggregate that further I need to breakdown the non visible minority group into 10 different population groups such as; black, South Asian, Arab etc… and then further disaggregate that by age and by sex or gender you start to tease apart all the data and that's where you find the serious issues that policy makers and programmers that program decision makers can address. Because you've now found the real issues that are hidden under the larger aggregated statistics.
Tegan: StatCan has to make decisions as an organization about which data to collect. What are some of the potential unintended consequences of collecting or not collecting different data?
Jeff: I'd like to go to an example again in police reported crime in Canada. So, right now we do not have information, so we have we do not collect information on the use of force in police services now, provincial governments or police services may, but at a national level. Statistics Canada does not yet collect information on the use of force, and we also don't collect information on the racial identity of individuals. Therefore, we are unable to say whether or not the use of force by police officers in Canada, is systematically more often used against racialized individuals, so our decisions do have an impact on what we can say. Now, That doesn't mean we're not trying to answer these questions, and there are many questions that we would love to answer, and sometimes we can't because the data doesn't exist. Sometimes we can't because we don't have the cooperation or buy-in of data providers yet, but. Every decision we make about what data we collect and what dating we don't collect, can have potential implications.
Tegan: Looking ahead, what can we do to get a more nuanced picture of race in Canada?
Jeff: So, when we talk about race again, it's it. I like to think about it as a social construct. So in that regard, do we have the right social constructs? Are we sure that our variables or our questions where we're trying to get a proxy for race, right? Because it's not necessarily, we're not asking the individual their DNA or their biological identity. We're asking them how they identify in society and maybe those need to change over time and So what we do is Statistics Canada. We have ten groups right now that we app that we consider to be generally covering it, but we always have to review those on a consistent basis and we're always looking at our standards our data standards to ensure we're actually, you know, reflecting society at large. And so we update those, we take the time to speak with Canadians. We do a lot of engagements and consultations to say, do these categories represent you effectively? We test the questions. If we make any changes, and so there's a very detailed process, but the key point here is that we want to make sure that we're consistently representing society, and so that's why it's critical.
Tegan: Analyst Filsan, again.
Filsan: I think what we're starting to realize is that it is very important to have diverse groups of people working in the field of data. Because again, I think the types of questions that we explore, we want to explore the type of questions and the different perspectives. That are offered really depends on having a diverse group of people being around the table doing you know the research, the data collection and the content determination process being involved in that. Being in decision making positions to propose different perspectives and to fill data gaps. I think that what people are starting to realize as well in terms of the whole objectivity thing, right? That, really, it's subjective right? what we have to realize is one way to account for biases, is to acknowledge that there is the subjective element in doing research, data collection, etc. Because we're all shaped by our experiences. Our you know education etc. And therefore if you have the diverse group of people around the table, you'll definitely, I think work towards filling different data gaps because your offer different perspectives and. And I think that's one thing that's being acknowledged more and more.
Tegan: What role could data play to stop the perpetuation of systemic racism?
Jeff: The use of data in, in tackling or addressing systemic racism is so critical if we think about it. Without understanding the phenomenon we're trying to address, it's very difficult to target policies and programs and legislation that will hopefully alleviate the issue. So, if you do not understand the nature of the problem, it's quite difficult to identify the solution. And so data provides us with two things; if we did not have the census in Canada, we would not be able to ascertain the composition of the entire country, and we need that first and foremost, because if we don't have that, we can't understand if there are inequities. So, let me think, if you think about the top CEOs in Canada, if 5% of those top CEOs are black, Is that reasonable or not? Without knowing the proportion of Canadian society that's black, you don't know. So if 5% of the Canadian society is black, that's a very reasonable representation. If 25% of this society is black and only 5% of your CEO's, now we have a discrepancy and data is the only way to really identify those critical issues.
Filsan: I think you know two things that are very important that you'll hear often is accountability and transparency. Because a lot of the experiences in systemic racism. One thing about it is it's very covert, right? It's hidden, it's not something that is seen. It's embedded in institutions, and therefore I think what data does is makes it visible. There's a lot of qualitative research that exists on systemic racism, and partly for the reason that it's very complex to kind of study identity in systemic racism. It's something that's very complex and therefore you have a lot of data that exists, and what official statistics or statistics does is it, I think it shows you the prevalence of an issue, right? There's more evidence that is built and those data gaps that exist are filled and therefore what you do have is accountability, right? Once you have the evidence and the data. Uh, people can use it and you have to do something about it. People can advocate and say, you know, here's a data to prove that this exists, or there's evidence that systemic racism exists, its prevalent, and therefore we want something the government to act, or a policy to be implemented. So I think those are two things that it does have. It does help, right? Yeah, accountability and transparency.
Tegan: Are there any particular endeavors or projects related to our discussion that you'd like to take the opportunity to highlight?
Jeff: One key project that the federal government has funded in 2021. Is the disaggregated data action plan and Budget 2021 Statistics Canada was given the single largest investment in over 30 years to try and tackle some of these systemic issues around identifying racism and gender gaps in society. This is a critical project. It's going to allow us to work with all of our partners across the country to better collect data that allows us to disaggregate and tell those critical stories about, you know, the inequities that we can uncover without these disaggregated data, it's quite challenging. And so this initiative is so critical, we are spending a lot of time and energy discussing this with the country, with provinces, with territories with our partners, with Canadians, with indigenous groups with racialized, groups were trying to spend the time to really understand how we should be collecting these data. How we should be disaggregating? How should we be reporting this? That's really fair and that is, you know, respectful, and so this project, I think, is a cornerstone of the way in which Statscan shifting its entire culture. This is not a project around a single subject matter like labour or justice or health. This is a fundamental shift in the way in which Statistics Canada is approaching data. So I think it is really important to highlight that project.
Tegan: If someone would like to learn more about disaggregated data and some of the work that STATCAN is doing, where can they find that?
Jeff: The best places on this Statistics Canada website. We actually have a wonderful area for disaggregated data action plan and there's a great infographic that explains what we're trying to achieve and why it's so important for society.
(Theme)
Tegan: You’ve been listening to Eh Sayers. A special thank you to Sarah, Filsan, and Jeff for taking the time to work with us on this episode. You can subscribe to this show wherever you get your podcasts. There you can also find the French version of our show, called Hé-coutez bien. If you liked this show, please rate, review, and subscribe. Thanks for listening!