Get involved in the DSN!
Become a member
Showcase your project
Meet the data scientist
Feedback survey
Data science resources
More information
The information in these articles is provided 'as-is' and Statistics Canada makes no warranty, either expressed or implied, including but not limited to, warranties of merchantability and fitness for a particular purpose. In no event will Statistics Canada be liable for any direct, special, indirect, consequential or other damages, however caused.
Recent articles
Applying Semi-Supervised Machine Learning Classification to Anomaly Detection Exercises: The Case of Sensor Data
Topics covered in this article: Computer vision, Predictive analytics
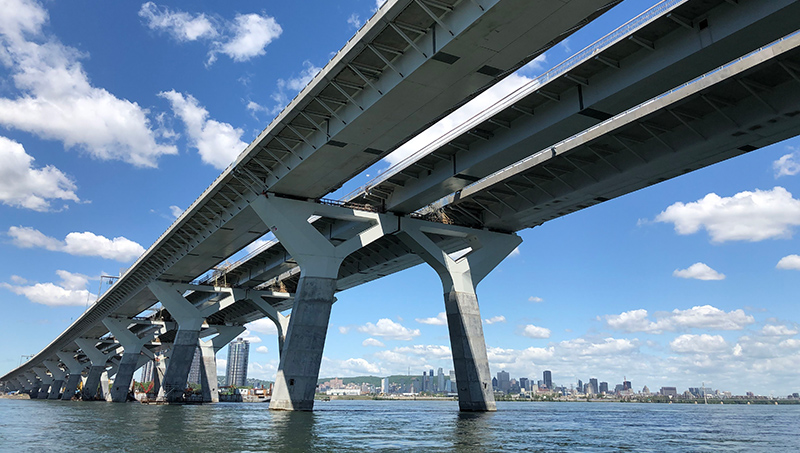
Source: Courtesy of Housing, Infrastructure and Communities Canada
Discover how the Data Science and Major Bridges and Projects teams at the Federal Government of Canada’s Department of Housing, Infrastructure and Communities (HICC) are revolutionizing bridge health monitoring. This article presents their innovative project that leveraged Machine Learning (ML) to filter noise from sensor data and enable precise monitoring of structural components. By combining ML classification with expert knowledge, the team successfully identified over 714,000 anomalies, and the results contributed to enhancing traditional monitoring methods. Learn about the techniques used and the significant impact on bridge maintenance. Don’t miss this insightful read on the future of infrastructure management!
Applying Random Forest Algorithms to Enhance Expenditure Predictions in Government Grants and Contributions Programs
Topics covered in this article: Ethics and responsible machine learning

This article showcases the successful collaboration between the Data Science team and the Grants and Contributions Centre of Expertise (GCCOE) at Housing, Infrastructure, and Communities Canada (HICC). Leveraging Machine Learning (ML), specifically the Random Forest algorithm, the project aimed to enhance expenditure forecasting for Grants and Contributions (G&C) programs. By integrating data-driven insights with financial expertise, the ML model significantly improved the efficiency and accuracy of the department’s G&C processes. This innovative approach was recognized with the Comptroller General of Canada Innovation Award in December 2024. The article highlights how ML can bolster financial stewardship, streamline operations, and provide a scalable framework for strategic planning across the federal government.
Data to Decisions: Visualizations and ML Modeling of Rental Property Data
Topics covered in this article: Data processing and engineering Computer vision
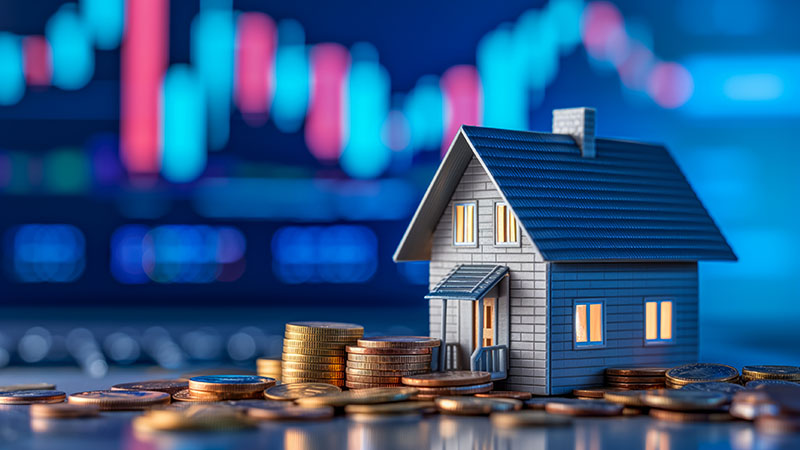
As per the 2021 census, there were 5-million rental households in Canada, which means roughly one-third of Canadian households are renters. However, much of this rental activity occurs privately, leading to limited and inconsistent data. To bridge this knowledge gap, NorQuest college, acquired processed, analyzed, and visualized rental listings from the stakeholder – Community Data Program, for Ontario.
Continue reading: Data to Decisions: Visualizations and ML Modeling of Rental Property Data
Other recent articles
Browse articles by topic
Computer vision
- Applying Semi-Supervised Machine Learning Classification to Anomaly Detection Exercises: The Case of Sensor Data
- Comparing Optical Character Recognition Tools for Text-Dense Documents vs. Scene Text
- Computer vision models: seed classification project
- Context modelling with transformers: Food recognition
- Data to Decisions: Visualizations and ML Modeling of Rental Property Data
- Extracting Temporal Trends from Satellite Images
- Greenhouse Detection with Remote Sensing and Machine Learning: Phase One
- Image Segmentation in Medical Imaging
- Indigenous Communities Food Receipts Crowdsourcing with Optical Character Recognition
- Reducing data gaps for training machine learning algorithms using a generalized crowdsourcing application
- Self Supervised Learning in Computer Vision: Image Classification
- Tackling Information Overload: How Global Affairs Canada's "Document Cracker" AI Application Streamlines Crisis Response Efforts
- The Rationale Behind Deep Neural Network Decisions
Data processing and engineering
- A new indicator of weekly aircraft movements
- Adopting a high Level MLOps Practice for the Production Applications of Machine Learning in the Canadian Consumer Prices Index
- An image is worth a thousand words: let your dashboard speak for you!
- Building an All-in-One Web Application for Data Science Using Python: An evaluation of the open-source tool Django
- Creating Compelling Data Visualizations
- Data Engineering in Rust
- Data to Decisions: Visualizations and ML Modeling of Rental Property Data
- Deploying your machine learning project as a service
- Designing a metrics monitoring and alerting system
- Extracting Public Value from Administrative Data: A method to enhance analysis with linked data
- Implementing MLOps with Azure
- Making data visualizations accessible to blind and visually impaired people
- MlFlow Tracking: An efficient way of tracking modeling experiments
- Non-Pharmaceutical Intervention and Reinforcement Learning
- The COVID-19 cloud platform for advanced analytics
- Writing a Satellite Imaging Pipeline, Twice: A Success Story
Predictive analytics
- Forecasting power consumption in remote northern Canadian communities
- From Exploring to Building Accurate Interpretable Machine Learning Models for Decision-Making: Think Simple, not Complex
- Modelling SARS-CoV-2 Dynamics to Forecast PPE Demand
- NRCan's Digital Accelerator: Revolutionizing the way Natural Resources Canada serves Canadians through digital innovation
- Unlocking the power of data synthesis with the starter gide on synthetic data for official statistics
- Use of Machine Learning for Crop Yield Prediction
Text analysis and generation
- A Use Case on Metadata Management
- Adopting a high Level MLOps Practice for the Production Applications of Machine Learning in the Canadian Consumer Prices Index
- Applied Machine Learning for Text Analysis Community of Practice: 2021 in review
- Bias Considerations in Bilingual Natural Language Processing
- Chatting About Chatbots: A review of the Chatbot Workshop
- Document Intelligence: The art of PDF information extraction
- Indigenous Communities Food Receipts Crowdsourcing with Optical Character Recognition
- Official Languages in Natural Language Processing
- Text Classification of Public Service Job Advertisements
- Topic Modelling and Dynamic Topic Modelling: A technical review
- Using data science and cloud-based tools to assess the economic impact of COVID-19
- Version Control with Git for Analytics Professionals
- 2021 Census Comment Classification
Ethics and responsible machine learning
- Applying Random Forest Algorithms to Enhance Expenditure Predictions in Government Grants and Contributions Programs
- A Brief Survey of Privacy Preserving Technologies
- Adopting a high Level MLOps Practice for the Production Applications of Machine Learning in the Canadian Consumer Prices Index
- Explainable Machine Learning, Game Theory, and Shapley Values: A technical review
- Identifying Personal Identifiable Information (PII) in Unstructured Data with Microsoft Presidio
- Introduction to Privacy-Enhancing Cryptographic Techniques
- Introduction to Cryptographic Techniques: Trusted Execution Environment
- Introduction to Privacy Enhancing Cryptographic Techniques: Secure Multiparty Computation
- Privacy enhancing technologies: An overview of federated learning
- Privacy preserving technologies part three: Private statistical analysis and private text classification based on homomorpic encryption
- Privacy Preserving Technologies Part Two: Introduction to Homomorphic Encryption
- Protected workloads on public cloud
- Responsible use of automated decision systems in the federal government
- Responsible use of machine learning at Statistics Canada
Other
- Production level code in Data Science
- Celebrating women and girls in science: An interview with Dr. Sevgui Erman
- Co-op student explores the power of Big Data
- Data Science Network Newsletter product feedback survey
- Developing Competency Profiles to Shape Data Science in the Public Service
- Developments in machine learning series: Issue three
- Developments in Machine Learning Series: Issue two
- Developments in Machine Learning Series: Series one
- First Data Science Network Directors' Committee Meeting
- Low Code UI with Plotly Dash
- Ottawa to hold World Statistics Congress in July 2023
- The Data Science Network newsletter turns one!